Algorithms Used to Justify Unfair Treatment of the Vulnerable
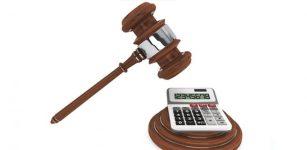
A highly-respected mathematician believes algorithms are being used to justify race and class bias by the police and courts.
Cathy O’Neil is a professor at Harvard University and author of the book ‘Weapons of Math Destruction’, which argues that the illusion of mathematical equations being objective and impartial is being exploited to justify the unfair treatment of minorities and the poor by the criminal justice system.
WMDs
These ‘weapons of math destruction’ have three key features: They are opaque, changeable and unfair.
Professor O’Neil explains that many mathematical algorithms aren’t actually objective, but are “models and opinions embedded in mathematics”.
She argues, for example, that the perception of minorities being prone to criminality is factored into the way police are deployed into communities, the way they deal with different groups, and even the court sentencing process itself.
Criminalising minorities
O’Neil’s book posits that sentencing legislation and resulting case-law typically contains aggravating factors which are far more prevalent in minority and other disadvantaged groups, and which, under an illusion of objectivity, ultimately justify the imposition of harsher penalties to members of those groups.
She argues that these factors often have far less to do with “justice” than ensuring that undesirable groups face the full force of the law, while those who live relatively affluent lives receive favourable treatment.
Factors such as prior convictions, prospects of rehabilitation, character, residence, associates and so on are analogous to parts of algorithms which work heavily against those who are brought up in disadvantages communities, and that far less emphasis should be placed on these factors when people are being sentenced.
“This is unjust,” O’Neil writes. “Indeed, if a prosecutor attempted to tar a defendant by mentioning his brother’s criminal record or the high crime rate in his neighborhood, a decent defense attorney would roar, ‘Objection, Your Honor!'”
O’Neil argues that while a judge might not always refer to such factors during the court process, they are nevertheless contained either explicitly or by inference in their judgments.
These same algorithms, O’Neil argues, are similarly used to predict areas which are more susceptible to crime. Higher numbers of police are deployed to poorer suburbs – normally inhabited by minorities and the poor – under this ‘predictive policing’ model, which leads to over policing, a higher rate of confrontations, a larger number of arrests and charges etc – making the areas seem more crime ridden than they actually are and, in the process, unfairly demonising vulnerable groups.
Crime statistics
The professor is highly critical of crime statistics, which she argues is largely a product of assumptions about criminality and over policing. She points out that this data is usually collated by police, who are more inclined to be deployed in large numbers to minority areas and arrest people in these areas.
Ms O’Neill gives the example of drug use. While research consistently suggests that the use of illegal drugs is spread almost equally across racial and income groups, police are overwhelmingly deployed to minority areas to enforce the law – resulting in a higher rate of arrests in these areas. By contrast, affluent areas are largely left alone.
She argues that if the algorithms used by police in their deployment were truly neutral, arrests for drug offences would be more equal across geographical locations.
O’Neill believes the system is “intrinsically biased.” “Police are basically sent back to the same neighbourhood where they’re already over policing. And in particular they’re not sent to neighbourhoods that have crime but where crimes aren’t found”.
Biased algorithms
Researchers from the independent investigative journalism site ProPublica analysed a program of predictive policing implemented in Florida, finding that it routinely rated blacks at a higher propensity of offending than whites.
The investigation found that only 20% of those classed as likely to commit crimes actually fell into that category, and that the program was inherently biased.
ProPublica concluded that the program’s algorithm was only “somewhat more accurate than the flip of a coin,” with 61% of those predicted to commit another crime going on to do so.
“The formula was particularly likely to falsely flag black defendants as future criminal, wrongly labeling them this way at almost twice the rate as white defendants,” the investigation reported.
It proceeded to find that white defendants were “mislabeled as low-risk more often than black defendants.”
The research raises questions about the fairness of current sentencing and policing regimes, provoking thought about whether current policies are about ‘blind justice’ or a way to justify the unfair treatment of vulnerable groups.